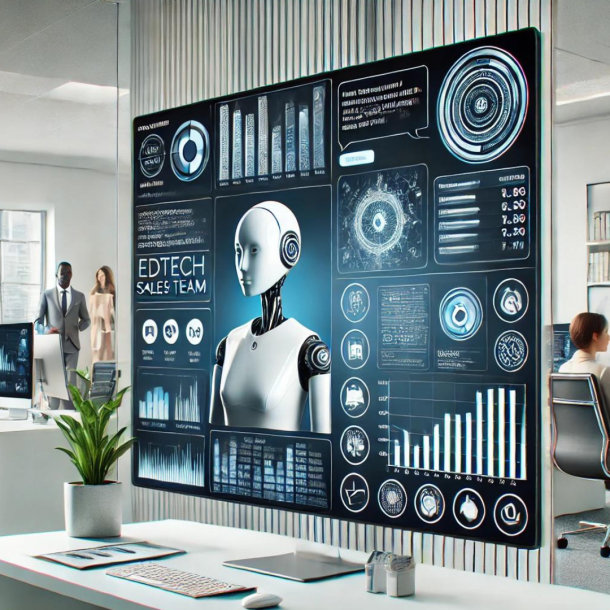
In recent years, AI-driven forecasting models have gained widespread use in businesses due to their ability to process large datasets and uncover patterns that traditional methods miss. Neural networks, machine learning, and deep learning are replacing intuition and basic statistics, providing more precise forecasts across industries.
According to a report by Salesforce, organizations adopting AI-driven forecasting have reported a 6.1% average increase in revenue and a 5.6% rise in profitability.
As AI continues to transform the business world, it’s clear that companies using these forecasting tools are better equipped to adapt to shifting trends and market dynamics. Embracing AI can give you a competitive edge in making data-driven decisions.
So, how exactly is AI changing traditional forecasting methods? And what are the outcomes and challenges of integrating these advanced tools into your ventures? Read on to find out how these AI-powered tools can reshape your forecasting techniques and practices.
Traditional sales forecasting is a systematic approach used by businesses to estimate future sales revenue based on historical data and predefined statistical models. This methodology typically employs techniques such as linear regression, autoregressive integrated moving averages (ARIMA), and exponential smoothing.
Despite its foundational role in business planning, traditional forecasting faces several challenges. It is prone to human error due to reliance on manual calculations and subjective interpretations, which can lead to significant deviations from actual outcomes. As businesses grow and data volumes increase, these methods can become cumbersome and less reliable, prompting a need for more advanced solutions that can enhance accuracy and adaptability in sales predictions.
AI forecasting is the use of advanced machine learning algorithms, such as neural networks and deep learning, to predict outcomes based on real-time and historical data. Unlike traditional methods, AI can process vast datasets, recognize complex patterns, and make accurate predictions faster. The key benefit is its ability to continuously learn and adapt to new information, making your forecasts more precise and actionable.
For example, AI doesn’t just rely on past data trends; it can factor in external variables like market conditions, seasonality, and customer sentiment. This makes your forecasts far more reliable and helps you stay ahead in fast-changing environments.
Transitioning from static forecasts to AI-powered models allows businesses to stay agile in an unpredictable market. But how exactly does AI outshine traditional methods?
You’re probably used to traditional forecasting, where data is manually collected and analyzed using basic statistical tools. Here’s how AI changes the game:
Traditional forecasting methods often struggle when it comes to scaling. As your business grows, the data you collect multiplies, but older methods typically rely on manual input or simple statistical models that can’t efficiently handle vast datasets.
AI, on the other hand, thrives on data. It can manage, process, and analyze large amounts of information from various sources, including structured and unstructured data like website interactions or social media trends. This ability to scale makes AI a far more versatile tool for growing businesses.
Traditional forecasting is slow, often requiring manual updates and complex calculations, which can delay decision-making. AI operates much faster by processing data in real-time.
For example, if market conditions shift suddenly or customer behavior unexpectedly changes, AI can instantly update forecasts to reflect those changes. This speed is essential in dynamic industries like retail, finance, or supply chain management, where even minor delays can lead to missed opportunities or financial loss.
The accuracy of traditional forecasting methods is often limited by the quality and quantity of the data, as well as the human input involved. AI improves accuracy by recognizing intricate patterns and trends across multiple datasets.
For instance, AI can analyze customer behavior alongside market conditions and product performance to make far more precise predictions. This higher level of accuracy is especially beneficial in industries with high volatility, where small forecasting errors can lead to significant miscalculations.
Also read: How Generative AI Can Completely Automate Your Sales Enablement Training: Benefits and Best Practices.
Now that you understand how AI differs from traditional forecasting let’s take a closer look at why AI can completely replace older methods. It’s not just about speed and accuracy. It’s also about scalability, bias elimination, and real-time responsiveness.
Traditional forecasting methods require constant human oversight and manual adjustments. Conversely, AI automates the entire process and learns from every piece of new data. This reduces human error, increases efficiency, and allows your business to respond to market changes more effectively.
AI forecasting is a dynamic field, offering a host of approaches to cater to diverse data sets and prediction requirements. Let’s explore the various AI-driven forecasting techniques to help you select the most suitable for better forecasting results for your business.
When it comes to AI, not all forecasting techniques are the same. Neural networks, deep learning, and AI-enhanced time-series models go beyond simple trend analysis.
These AI-driven models identify and adapt to patterns in real-time, allowing businesses to make more precise predictions. Curious about which techniques might work best for your business? Let’s break down how each one works.
Neural networks are designed to mimic how the human brain processes information, but they do it much faster and with more data.
This subset of AI can process huge volumes of data, identifying patterns and insights that aren’t immediately obvious to human analysts.
Traditional time-series analysis is based on past data, like sales or stock prices over time. AI-enhanced time-series models take this a step further by integrating real-time data and external variables such as economic conditions or market trends. This leads to more dynamic, accurate forecasts, especially in industries where historical patterns alone aren’t enough to predict future outcomes.
At this point, you may ask, “What are the practical benefits?” AI forecasting doesn’t just promise better accuracy. It transforms how you allocate resources, react to market changes, and optimize operations. Let’s explore the business outcomes that AI prediction can bring to your venture.
A LinkedIn collaborative article collection features several articles on forecasting with expert comments from the LinkedIn community. Many of the articles have comments from leading experts in the AI industry. The collection offers articles on forecasting, from basic understanding to analysis to complex AI forecasting algorithms.
By moving beyond manual data entry and into predictive, real-time analysis, AI empowers businesses to make faster, smarter decisions. Let’s examine how this can significantly improve business outcomes.
One of the biggest advantages AI offers is speed. By providing real-time updates, AI allows your business to react faster to market changes. Whether it’s a sudden surge in demand or an unexpected supply chain issue, AI helps you adjust your strategy immediately, ensuring that opportunities aren’t missed and risks are mitigated early.
AI doesn’t just predict demand; it helps you allocate resources more effectively. With accurate, real-time forecasts, you can optimise inventory levels, staffing, and production schedules to match predicted demand. This reduces waste and ensures that you’re always prepared to meet customer needs without overextending your resources.
Forecasting with AI significantly reduces the need for manual data processing and analysis, freeing up valuable time and labour. This allows your team to focus on more strategic tasks, driving productivity and reducing costs associated with human errors or outdated processes. Furthermore, better forecasting reduces the costs of overproduction, stockouts, or underutilization of resources, which directly affect your bottom line.
AI’s predictive power has practical applications that extend across various sectors, transforming how companies operate. Let’s explore how AI is being applied in real-world settings.
AI is more than just a theory. Many industries are already seeing its benefits. From retail to finance, businesses use AI forecasting to refine inventory management, optimize supply chains, and improve financial planning.
EXAMPLE: Retailers are increasingly adopting AI forecasting to enhance inventory management and sales predictions. Companies like Walmart utilise AI-driven models to analyze customer purchasing patterns, ensuring that products are stocked appropriately across various locations. This not only minimizes waste but also maximizes sales opportunities by aligning inventory with consumer demand.
Of course, no technology comes without challenges, and AI forecasting is no exception. Understanding these challenges will help your transition to AI forecasting more smoothly. Here’s what you should consider before fully adopting AI.
While the benefits are substantial, you’ll need to ensure data quality and be mindful of implementation costs. And while AI can do a lot, human oversight is still necessary to interpret its predictions effectively.
AI forecasting offers a clear path forward for businesses that need accurate, scalable, and real-time insights. While traditional methods are slow and prone to error, AI processes data faster, predicts trends more accurately, and scales effortlessly as your business grows. As you move toward a future with AI-driven forecasting, you’ll be better equipped to make smarter, faster decisions that will keep you competitive in any market.
Zipteams offers several features tailored for sales forecasting. The AI-powered sales tools feature conversational and predictive analytics for better engagement in your sales calls. With intent scoring based on customer interactions and advanced analytics dashboards, these tools allow sales teams to predict outcomes by analysing call and meeting data.
Additionally, sales pitch quality scores and training automation ensure ongoing improvement, which supports more accurate forecasts.
With real-time customer insight on sales calls, Zipteams helps you:
Boost your sales performance with Zipteams and stay ahead of your goals with precision forecasting tools designed for modern teams.
Cookie | Duration | Description |
---|---|---|
cookielawinfo-checkbox-analytics | 11 months | This cookie is set by GDPR Cookie Consent plugin. The cookie is used to store the user consent for the cookies in the category "Analytics". |
cookielawinfo-checkbox-functional | 11 months | The cookie is set by GDPR cookie consent to record the user consent for the cookies in the category "Functional". |
cookielawinfo-checkbox-necessary | 11 months | This cookie is set by GDPR Cookie Consent plugin. The cookies is used to store the user consent for the cookies in the category "Necessary". |
cookielawinfo-checkbox-others | 11 months | This cookie is set by GDPR Cookie Consent plugin. The cookie is used to store the user consent for the cookies in the category "Other. |
cookielawinfo-checkbox-performance | 11 months | This cookie is set by GDPR Cookie Consent plugin. The cookie is used to store the user consent for the cookies in the category "Performance". |
viewed_cookie_policy | 11 months | The cookie is set by the GDPR Cookie Consent plugin and is used to store whether or not user has consented to the use of cookies. It does not store any personal data. |