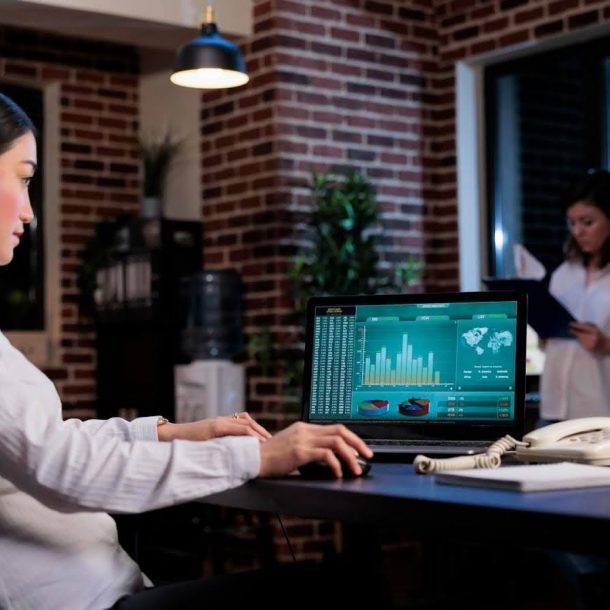
Revenue forecasting is one of the most crucial practices for any business. It provides the foundation for informed decision-making, financial planning, and strategic growth. Accurate forecasts allow companies to anticipate cash flow needs, allocate resources effectively, and set realistic sales targets—all critical components for sustainable business growth.
However, traditional forecasting methods now face limitations as customer behaviours, market dynamics, and the sheer volume of data have evolved significantly. Customer management has shifted towards personalised engagement, making it necessary to incorporate real-time insights into forecasting. Meanwhile, unpredictable market trends and the overflow of data from digital channels have created a need for advanced technologies to process, analyse, and rapidly adapt forecasts.
This is where AI, particularly generative AI, comes into play, offering a new horizon in revenue forecasting. AI-driven tools bring powerful capabilities, such as real-time adaptability, multi-scenario simulations, and pattern recognition, allowing companies to create more accurate, responsive forecasts. In this blog, we’ll explore how generative AI is shaping the future of revenue forecasting, uncovering its transformative features and the competitive edge it brings to financial planning.
Revenue forecasting involves predicting future revenue based on data from sales pipelines, historical sales data, market dynamics, and economic indicators.
Accurate forecasting supports strategic financial planning, resource allocation, and operational efficiency, helping businesses prepare for future cash flow needs and make informed decisions.
An accurate revenue forecast can be the foundation of sustainable growth and resilience. Inaccurate or outdated forecasts can lead to missed opportunities, over- or under-spending, and inefficient resource allocation.
Traditional revenue forecasting methods rely heavily on historical sales data, past market demand, and relatively simple trend analysis. These static models, while valuable, struggle with the fast-evolving market landscape, including unpredictable customer demands, sudden economic shifts, and fluctuating supply chains.
Key Limitations of Traditional Forecasting:
As companies scale, customer behaviour, sales volumes, and data complexity grow, making accurate forecasts with traditional models becomes challenging. This is where generative AI can drive accuracy, adaptability, and resilience.
Now that we’ve set the stage, let’s look at how AI is transforming these challenges into opportunities.
Generative AI steps in to bridge these gaps by enhancing forecasting with predictive analytics, real-time data processing, and scenario generation, making it a valuable asset for business intelligence and revenue management.
Here’s what AI brings to the table in revenue forecasting:
Generative AI enables data acquisition and preparation from diverse sources like sales performance, customer interactions, and external market data. It enriches traditional data sets with synthetic data when real data is insufficient, improving the forecast’s robustness.
Example: In retail, generative AI can leverage both historical sales data and synthetic customer behaviour data to create more realistic demand forecasts, helping retailers manage inventory efficiently.
One of generative AI’s most powerful capabilities is multi-scenario simulation. This AI can create “what-if” scenarios that help businesses test revenue predictions under different market dynamics or consumer trends, empowering them to adapt strategies quickly in response to sudden market shifts.
Generative AI models learn from evolving data, meaning they can continuously update forecasts in real-time. As new data points enter the system, AI-powered revenue prediction adjusts automatically, enabling data-driven revenue insights that reflect current market conditions.
AI’s automated anomaly detection can identify irregularities in revenue trends, alerting teams to deviations from expected patterns. This feature is crucial in spotting early signs of potential financial risks, such as sudden drops in sales or unusual increases in operational costs, and supporting sustainable business growth.
Example: A financial service provider can use anomaly detection to flag sudden dips in revenue due to regulatory changes, allowing the company to adjust its forecasts and client strategies accordingly.
For businesses that have established forecasting practices, AI offers a timely update to the ongoing system. Let’s explore how AI boosts the existing revenue forecasting practices.
For companies already utilising revenue forecasting, generative AI enhances their existing frameworks by enabling faster, more flexible, and more accurate projections. By integrating generative AI with traditional forecasting models, businesses can make more data-driven revenue insights accessible across teams, allowing for collaborative, informed decision-making.
Key Enhancements Generative AI Brings to Traditional Forecasting:
Revenue forecasting and its benefits differ from business to business. AI can be applied in various revenue forecasting practices to extract the most potential benefits of AI in revenue forecasting. Below, we explore industry-based applications of AI in revenue forecasting.
Generative AI’s versatility allows for tailored applications across industries, each with unique data needs and market dynamics. Here’s how generative AI benefits various sectors:
Industry | Generative AI Applications | Benefits |
Retail | Predicting seasonal demand and managing inventory efficiently | Reduces stockouts and overstocking, optimises revenue based on consumer trends |
SaaS | Churn prediction, subscription renewals, retention strategy optimisation | Stabilises recurring revenue and supports customer retention |
Manufacturing | Supply chain forecasting, revenue impact of production timelines | Ensures supply chain resilience, enables proactive revenue adjustments |
Each industry benefits from the data-driven insights and proactive adjustments generative AI enables, fostering business sustainability in uncertain markets.
Generative AI’s benefits are not limited to accurate forecasting, and rather AI can work as a round-a-clock assistant who provides reliable and objective predictions. Up next, we will break down how AI boosts the credibility of its forecasting.
Generative AI doesn’t just improve accuracy; it also enhances resilience. In uncertain economic climates, companies that rely on traditional forecasting methods often struggle to pivot quickly. Generative AI offers a solution through:
With the ongoing development of AI technology, the future of revenue forecasting is more promising than ever. Let’s break down what AI holds for the future of revenue forecasting.
As generative AI continues to advance, its role in revenue forecasting will only grow. Here’s a glimpse into the future of AI-driven forecasting:
Companies that start integrating generative AI into their forecasting processes now will be better prepared for this evolving landscape, equipped to make faster, more accurate, and resilient financial decisions.
Zipteams leverages conversational AI to transform customer interactions into actionable insights, significantly enhancing revenue forecasting through real-time data on customer intent, engagement, and sentiment. Here’s how Zipteams’ key features enable precise forecasting:
With Zipteams, companies gain unparalleled accuracy in revenue projections by aligning financial expectations with real customer engagement data, optimising sales efforts, and improving team productivity.
Book a demo today and make super accurate revenue forecasting for your business growth.
Cookie | Duration | Description |
---|---|---|
cookielawinfo-checkbox-analytics | 11 months | This cookie is set by GDPR Cookie Consent plugin. The cookie is used to store the user consent for the cookies in the category "Analytics". |
cookielawinfo-checkbox-functional | 11 months | The cookie is set by GDPR cookie consent to record the user consent for the cookies in the category "Functional". |
cookielawinfo-checkbox-necessary | 11 months | This cookie is set by GDPR Cookie Consent plugin. The cookies is used to store the user consent for the cookies in the category "Necessary". |
cookielawinfo-checkbox-others | 11 months | This cookie is set by GDPR Cookie Consent plugin. The cookie is used to store the user consent for the cookies in the category "Other. |
cookielawinfo-checkbox-performance | 11 months | This cookie is set by GDPR Cookie Consent plugin. The cookie is used to store the user consent for the cookies in the category "Performance". |
viewed_cookie_policy | 11 months | The cookie is set by the GDPR Cookie Consent plugin and is used to store whether or not user has consented to the use of cookies. It does not store any personal data. |